Abstract
Electrochemical impedance spectroscopy (EIS) is a characterization technique used widely in electrochemistry. Obtaining EIS data is simple when modern electrochemical workstations are used; however, analyzing EIS spectra is still a considerable quandary. The distribution of relaxation times (DRT) has emerged as a solution to this challenge. Nevertheless, DRT deconvolution underlies an ill-posed optimization problem, which is often solved by ridge regression (RR). RR’s accuracy strongly depends on the choice of the regularization level as encapsulated in the parameter λ. In this article, five cross-validation methods (i.e., generalized cross-validation, modified generalized cross-validation, robust generalized cross-validation, re-im cross-validation, and k-fold cross-validation) and the L-curve method are studied for the selection of λ. A hierarchical Bayesian DRT (hyper-λ) deconvolution method is also analyzed, whereby λ_0, a parameter analogous to λ, is obtained through cross-validation. The analysis of synthetic data suggests that the values of λ selected by generalized and modified generalized cross-validation are the most accurate among the methods studied. Furthermore, synthetic EIS spectra show that the hyper-λ approach outperforms optimal RR when λ_0 is obtained by generalized and modified generalized cross-validation. This research is expected to foster additional research on the central topic of regularization level selection for DRT analysis. This article not only explores various cross-validation methods, but also provides, through pyDRTtools, an implementation that may serve as a starting point for future research.
Content
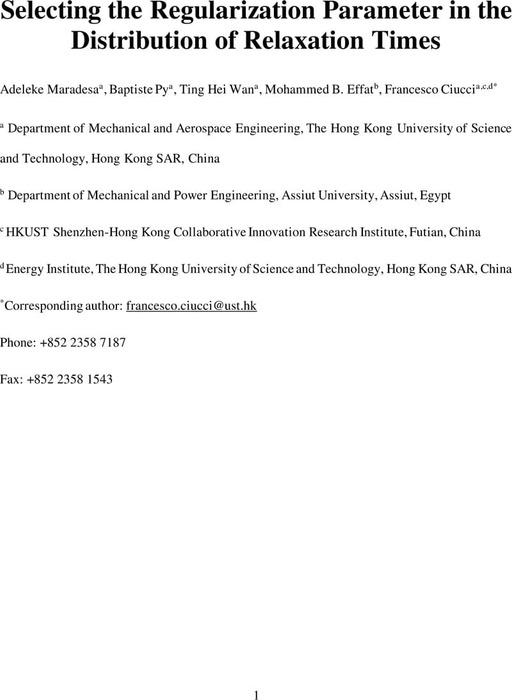
Supplementary materials
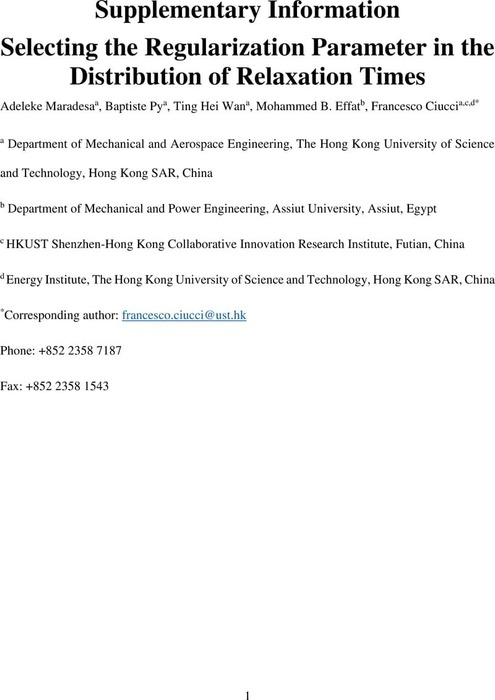
Selecting the Regularization Parameter in the Distribution of Relaxation Times
This supplementary material provides additional details to the main manuscript, this includes additional contents, tables, and figures.